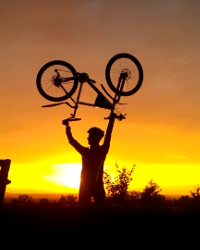
manfred dreese
Software Craftsman, Clean Coder, Senior Developer, Photographer and Cyclist << Personal Blog
Software testing generally raises an association with tests that determine the correctness of an implementation by asserting against an expected behavior or result. Regardless of their importance, tests on unit- and integration level cannot answer all questions regarding the production readiness of an implementation. Performance, resource efficiency and security become even more important in connection with cloud solutions, where more efficient code has a much higher likelihood of scaling better and saving costs.
Continue ReadingRestic is a slim and fast backup-solution tailored for users who prefer automation capabilities over a fancy user interface. It also supports off-site backups out of the box, using standard protocols such as SSH or even S3 compatible memory. Restic considers the storage as not trusted in terms of privacy, so the backup uses strong encryption, which comes in handy when using cloud providers. This article provides a brief instruction on how to use 1und1 IONOS Cloud S3 Object storage as a remote backend.
Continue ReadingI recently withness and participate in intensive debates regarding the superiority of either “serverless”/FaaS or containers in any form in terms of management and orchestration. Often, people are very vocal about an extreme position of the spectrum, while I pursue a much more relaxed, neither less opiniated approach. This post is supposed to briefly summarize my opinion. I will not dive deeply into the details of containers and FaaS more than neccessary to avoid redundancy and keep the post focused.
Continue ReadingIf you are currently preparing for an AWS Solution Architect certification, you might have stumbled across numbers of articles providing hints and experiences on how to pass the exam, often consisting of affiliate links and talking heads on video. In this article, I want to provide my point of view regarding preparation and passing the exam - completely ad-free. Preparation There are several ways of preparing for an AWS Solution Architect Associate (OK Google!
Continue ReadingA 10minutes video I made with the intent to capture the relaxed mood during a sunday walk on the island of Heligoland. Please keep in mind that the video is hosted on Youtube, so either be aware that they might set some cookies, or better: have your Adblocker armed!
Continue Reading